Human-centered Computing:
Learning Intelligent Analytics from Multimodal Data
What is the abstraction of human-centered AI research?
Imagine humans as complex dynamical systems: systems that are characterized by multiple interacting layers of hidden states (e.g., internal processes involving functions of cognition, perception, production, emotion, and social interaction) generating multimodal measurable signals (speech and language, physiology, gestures, facial expressions, etc.). This abstraction of humans with a signals and systems framework has already brought a synergy between engineering (e.g., signal processing and machine learning research) and behavior sciences (psychology, health, human resource, organizational research, security, etc.) and has sparked research effort in developing and applying human-centric computational methods across domains. Moreover, the abstraction also has positioned several emerging cross-cutting interdisciplinary research fields, e.g., affective computing, social signal processing, and behavioral signal processing for human-centered applications. The set of problems that human-centric computing researchers face is essentially that of identifying the hidden attributes of the system which reflects in the various realizations of signal and data measured and collected, uncovering through novel signal processing and machine learning on big data.
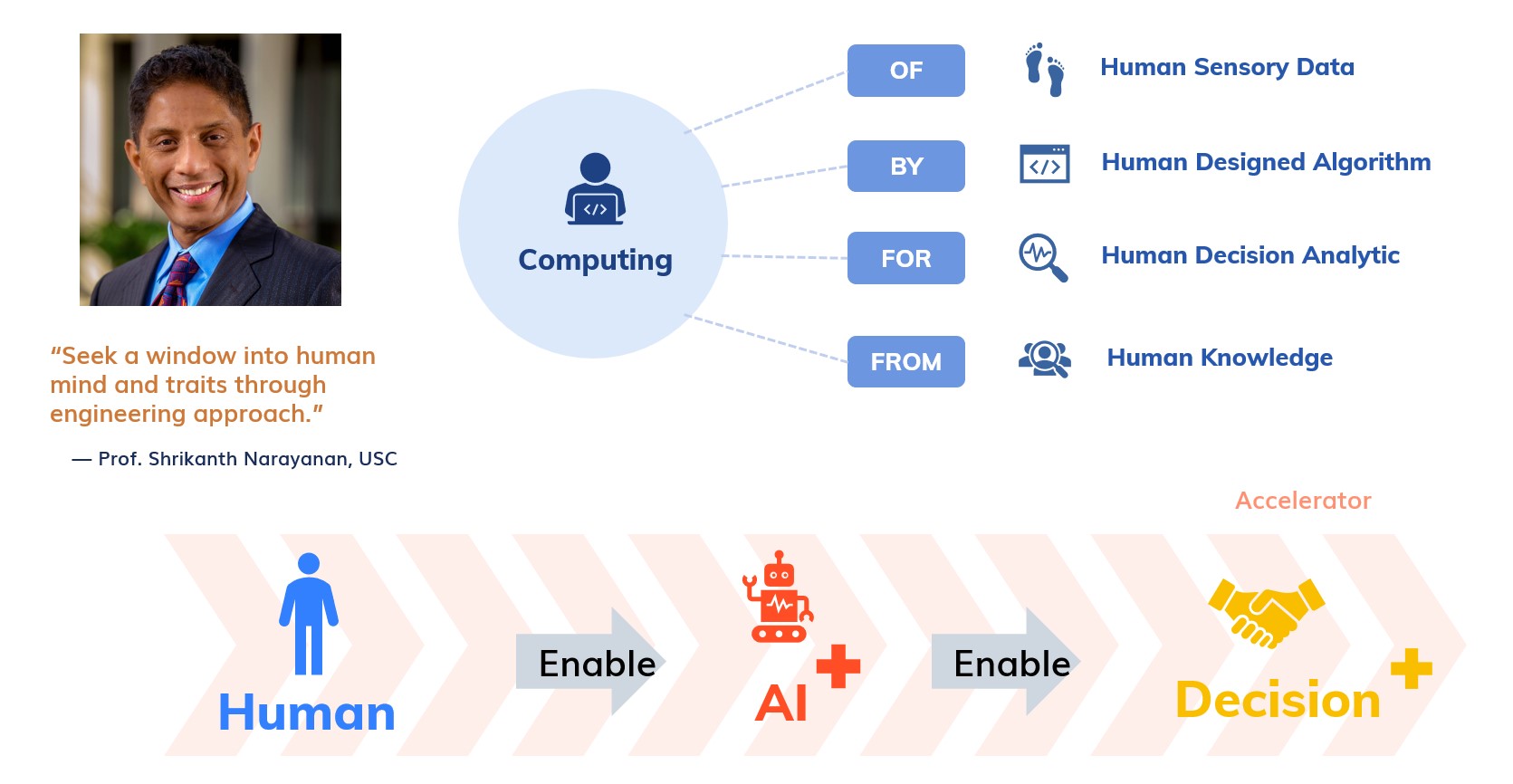
Why handling such a complex problem of humans with an engineering approach now?
The current era of data ecosystem continues to grow and become highly integrated into our daily lives, e.g., low-cost physiological wearables that can derive direct measures of a person's internal state are increasingly common, as are commodity vision and audio devices that collect expressive behavior data in large quantity with higher fidelity, moreover, the existing cyber-physical systems (e.g., web, cell phone apps, clinical system in hospital, and many other structured databases, etc.) are becoming relevant, integrative, and diverse enough to be considered as important footprints of the users. These large quantities of signal data with heterogeneous spatial-temporal resolution provide the necessary ingredients in learning human-centered analytics in a multimodal data-driven approach.
The potential market size for these human-centered applications is tremendous; for example, just for the medical Internet-of-Things (mIoT) market, it has been projected to be worth $117B USD by 2020, representing 40% of the total IoT market, and the use of signal processing and AI algorithms are expected to push that value even higher. Furthermore, Gartner's hype cycle for emerging technologies has put affective computing (2017), emotion AI (2019) onto the chart, and recently it has stated that the emotion AI technology by itself is at least a $20B USD industry. Many applications can benefit from emotion AI, e.g., intelligence emotion-aware customer service can serve better with care, HR system identifies candidates efficiently, and business persuasion could also be improved when training sales personnel. Numerous industries, even those beyond current imaginable service industries, can easily create a value-added service solution by applying this emotion detection technique specifically through behavior interaction services.
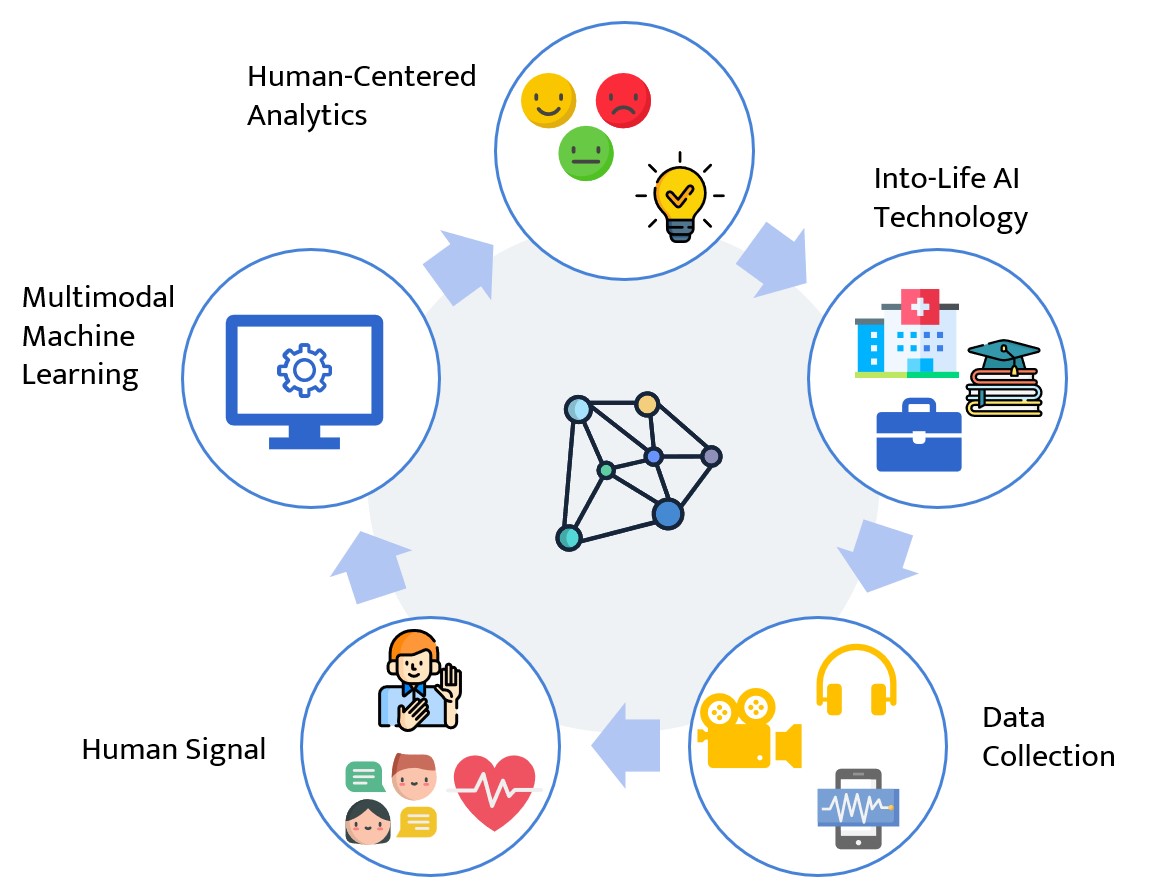
What is the next step in algorithmic development?
Being a relatively young research domain globally with the complication and challenges in modeling human behaviors, there remains a need to continuously develop AI-enabled behavior analytics. Specifically, the challenges lie in the complexities of modeling human behaviors – from typical to distressed and disordered manifestations – computationally with AI-enabled algorithms and in the contextualization of such analytics in their relevant realm of application domains. The complexities are centered on the issue of heterogeneity of human behaviors. Sources of variability in human behaviors originate from the differences in mechanisms of information encoding (behavior or physio generation) and decoding (behavior perception). An additional layer of complexity exists because human behaviors occur largely during interactions with the environment and agents therein. This interplay, which causes a coupling effect between inter-human behaviors, is the essence of unique dynamics that has been at the core of human communications. This dependency-induced dynamic creates intricate variabilities along with variable time scales, and across interaction contexts. Lastly, much of the research effort needs to be contextualized in a meaningful and domain-aware manner. This involves translating the knowledge into a range of domains (e.g., the arts, education, and healthcare) in order to create a tangible impact.